Model Optimization Techniques: LoRA
Discover how advancements like Low Rank Adaptation (LoRA) and Quantization are transforming AI models, making them more efficient and accessible to a broader audience.
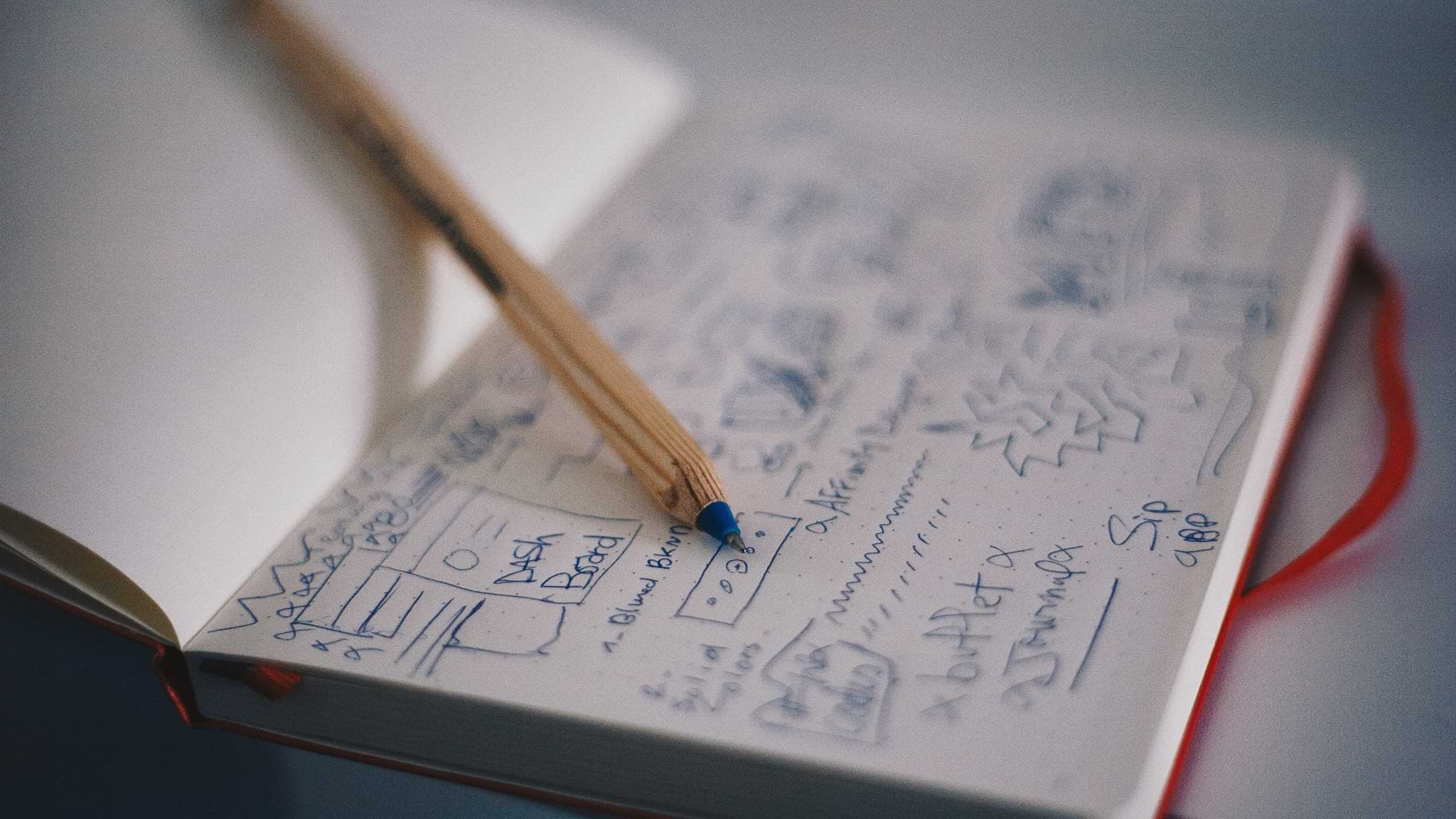
Advancements in AI are rapidly transforming the landscape, with new techniques such as Low Rank Adaptation (LoRA) and Quantization playing pivotal roles in making AI models more efficient and accessible. These innovations are not only enhancing model performance but also democratizing access to sophisticated AI capabilities.
Low Rank Adaptation (LoRA)
LoRA is a cutting-edge technique aimed at optimizing the performance of AI models. Instead of fine-tuning billions of model parameters directly, LoRA involves freezing the pre-trained model weights and injecting trainable layers. These layers represent the changes to model weights as two smaller, lower-rank matrices in each transformer block. This approach dramatically reduces the number of parameters that need to be updated, speeding up the fine-tuning process and reducing the memory required to store model updates.
LoRA's efficiency is particularly beneficial for smaller organizations and startups that may not have access to extensive computational resources. By lowering the barriers to entry, LoRA enables these entities to harness the power of advanced AI models without significant infrastructure investments.
Quantization
Quantization is another technique revolutionizing the field of AI. It involves reducing the precision used to represent model data points—for example, converting from 16-bit floating point to 8-bit integer. This reduction in precision lowers memory usage and speeds up inference, akin to lowering the bitrate of audio or video to reduce file size and latency.
Quantization, especially when combined with LoRA (as in QLoRA), can significantly enhance the performance and accessibility of AI models. It allows models to run more efficiently on less powerful hardware, making advanced AI capabilities available to a wider audience.
Customized Local Models and Data Pipelines
These advancements are also enabling enterprises to pursue differentiation through bespoke model development. Open source models and tools can be tailored to specific real-world scenarios, from customer support to supply chain management and complex document analysis. This customization is particularly relevant in industries with highly specialized vocabulary and concepts, such as legal, healthcare, and finance.
By developing models small enough to run locally on modest hardware, organizations can maintain control over their data and operations, enhancing both security and performance.
Application to iChain
At iChain, these model optimization techniques are integral to our mission of providing decentralized, user-controlled AI solutions. By leveraging LoRA and Quantization, we can offer powerful AI tools that are both efficient and accessible, even to users with limited computational resources. This ensures that our platform remains inclusive and capable of serving a diverse user base.
Our focus on customized local models and data pipelines allows us to tailor our AI solutions to the unique needs of our users, from personalized financial insights to advanced data privacy measures. These optimizations not only enhance the performance of our AI tools but also align with our commitment to decentralization and user empowerment.
Conclusion
The advancements in model optimization techniques like Low Rank Adaptation and Quantization are making AI more efficient and accessible. These innovations are breaking down barriers and enabling a broader range of organizations and individuals to leverage sophisticated AI capabilities. At iChain, we are committed to integrating these techniques to provide powerful, user-friendly AI solutions that drive innovation and inclusivity in the decentralized ecosystem.
Stay tuned for more updates as we continue to push the boundaries of AI technology at iChain.